Main Content
ModelForge
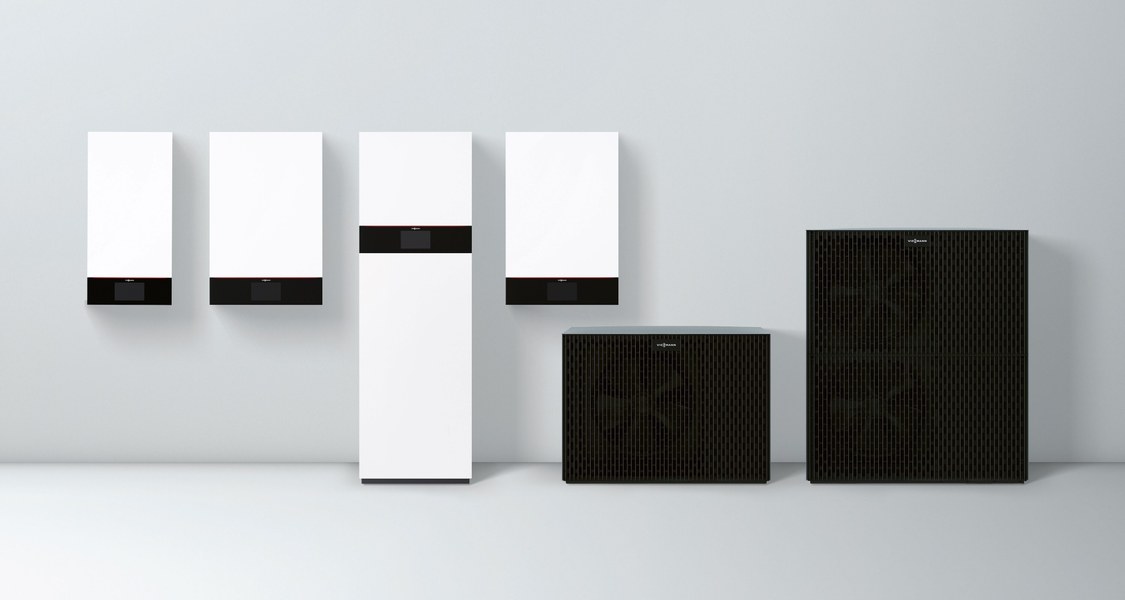
Many manufacturers of electrical installations in smart home environments have developed and now offer AI solutions that record and analyze the sensor data from their products. Their goal is to monitor and forecast runtime parameters, such as the energy consumption of heat generators or the cooling performance of air conditioning systems, for predictive maintenance and to optimize the carbon footprint. The training and deployment of such AI models can, though, be costly, necessitating intelligent techniques to consolidate, i.e., aggregate models of individual installations into fewer, but larger models. The aggregation of AI models, however, poses a challenging task due to the complexity of the systems and the variability of (hidden) factors that influence the forecasts. To solve the aggregation challenge, improve the forecasting accuracies and ultimately also reduce the AI deployment costs, this project explores the concept of consolidating similar machine learning models with a novel clustering approaches.
The first paper on the ModelForge project was presented at LWDA 2023.