Main Content
Uncertainty quantification
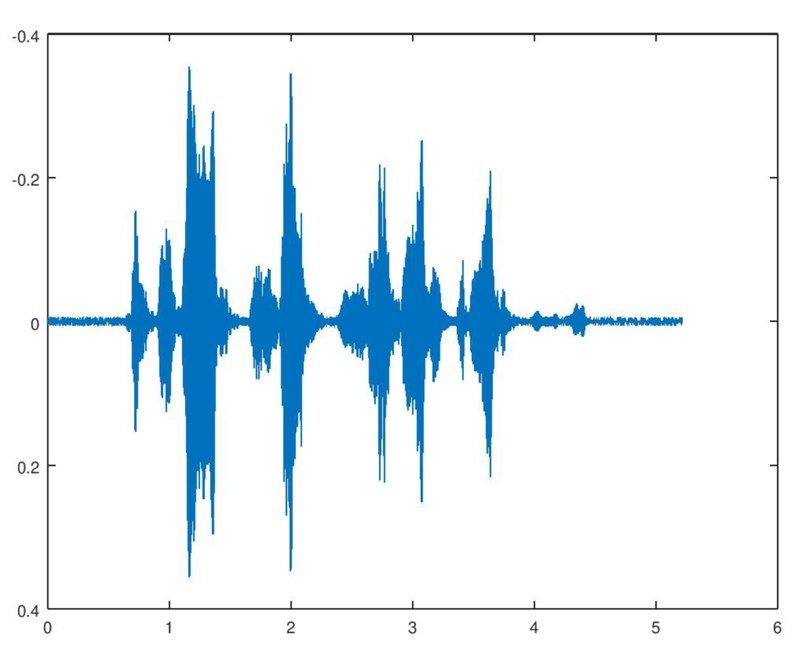
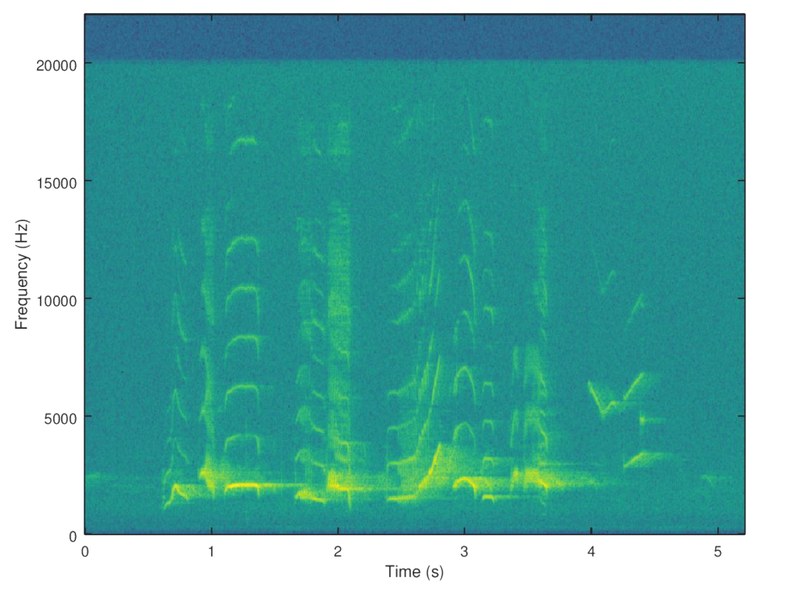
In the research field Uncertainty Quantification (UQ), uncertainties are considered in different application fields. These uncertainties usually result from the fact that some aspects of the system are not known exactly. Many models of real processes in science and engineering but also in life sciences are therefore subject to such uncertainties. In mathematics, the uncertainty is often modeled by a
probability distribution.
The central task of UQ is to quantitatively characterize the uncertainties, reduce them, and determine how likely certain outcomes are under the given uncertainties.
In the analysis of the forward propagation of uncertainties, discrete and continuous parameters of physical laws are modeled stochastically. The mathematical task is to quantify the uncertainty in the solutions resulting from the model by mathematical quantities and structures in order to be able to make reliable statements about the modeled processes.
In inverse UQ, on the other hand, the parameters of a mathematical model, which is to describe the system, are to be reconstructed from noisy observations of a system and their uncertainty quantified. This class also includes mathematical problems of machine learning and combinatorial algebra.
Simulations from the model can be contrasted with observations for a reduction in modeling bias.
Central aspects of both problems - forward and inverse problems - require a combination of numerical, stochastic and combinatorial methods as well as methods of optimization for their solution. This dovetailing of methodology requires a manifold bilateral and multilateral interaction of the numerics/optimization, stochastics and discrete mathematics research groups. In addition, the rapid progress in Machine Learning and Data Science in recent years has become one of the rich sources of challenging problems in UQ theory and practice and has closely linked the field to the corresponding computer science groups.