Main Content
Big Data and AI in biodiversity research
How can we reliably use 300 year old herbarium specimen for modern biodiversity research?
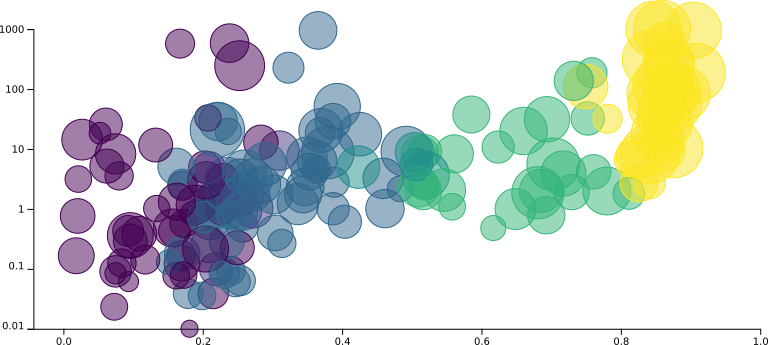
The public availability of biodiversity data is drastically increasing. For instance information of species geographic occurrence from digitized herbarium collections, molecular data on species relationships from high throughput sequencing, and information on species traits, from mobilized data in floras. Currently methods to process “big data” are a bottleneck for harnessing the full potential of large datasets and transitioning biodiversity research into a data driven discipline. We develop software methods for standardized and reproducible analysis of large-scale biodiversity data with a focus on georeferenced species occurrence records mobilized from collection records. Among other we develop CoordinateCleaner, an R-package to quality control large scale species occurrences from collections (Zizka et al., 2019), SampBias, an R-package to quantify geographic sampling bias in occurrence records (Zizka et al. 2021) and Bio-Dem, a graphical user interface tool to visually link scientific collection activity to socio-political conditions in countries worldwide, including colonial history (Zizka et al. 2021, 1st prize at the 2021 GBIF Ebbe Nielsen Challenge)
Automated Red Listing using Artificial Intelligence
How can we conserve as much plant diversity as possible for future generations?
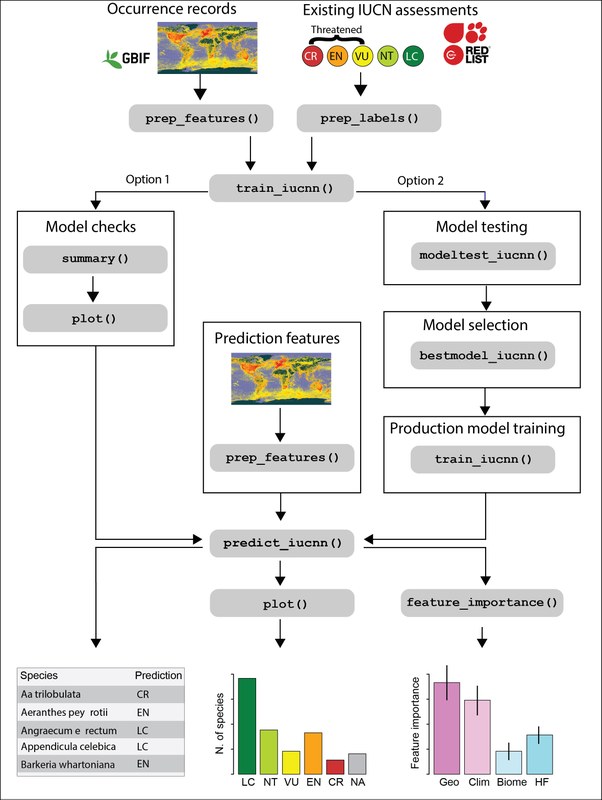
Effective and engaged conservation is essential in the face of the ongoing biodiversity crisis. Red Lists of threatened species quantify species extinction risk and are a key tool in conservation research, practice and communication. Yet, due to the time-intense red-listing process, most Red lists are biased towards well-studied taxa (mammals, birds) and regions and temperate regions) while only a fraction of other groups such as plants or soil organisms are evaluated, meaning that important conservation decisions miss key components of biodiversity. We develop automated conservation assessments that use machine learning approaches to approximate species Red List status based on information from biological collections, floras and remote sensing data. In collaboration with Researchers from other European institutions we develop algorithms and software applying large datasets and deep learning to approximate species extinction risk in a Red List framework (Zizka et al. 2022). Recently we have applied this approach to quantify the extinction risk of orchid species worldwide (Zizka et al. 2022).